As I said before, EVERY COMPANY MUST be a tech-driven company. This new tech landscape is driving the hyper-growth stories we are seeking here, as companies sprout up to be the "picks and shovels" plays that are supplying this gold rush of technological innovation. They are creating new tools that are enabling companies to solve their own problems themselves, that are cross-applicable to every business, across every industry. And luckily for us, it still seems like the early innings!
I have spoken on MDB before, and haven't felt the need to dive very deep into the technical details of their product line, as it seems pretty easily understood -- once you know what a NoSQL document store is, you know what MongoDB excels at. But let's walk through their history a bit and where it has put them strategically. [Reminder, I call the company MDB to differentiate product from company. Elastic thankfully makes it easier so I refer to them by name. Downside is this means I use the word "elastic" over 200 times here.]
MDB started by making an open-source NoSQL database, then it sold support and tooling for that database to enterprises that were using it for either their internal database or as an embedded database within their products. Once cloud computing took hold, MDB then started providing a managed, vendor-neutral, cloud hosting service for its core database... one that its customers flocked to, for its scale, high availability (HA), ease of use, and the fact it completely saves them money by eliminating costs around infrastructure and ongoing maintenance. MDB's approach has them creating a core platform around MongoDB of tools that reduce customer friction -- for either self-hosted or for managed Atlas. They have apps for data exploration (Compass) and a mgmt interface (Ops Manager or Cloud Manager). They have SaaS tooling around Atlas service, like a serverless platform (Stitch), a cloud migration tool, and a visualization dashboard tool (Charts, in beta). And as I discussed before, it's now increasing customer flexibility and increasing the applicability of its platform with its moves into being a synchronized mobile database (also in beta, but, finally, with a major acquisition to help them move faster).
Elastic is an incredibly similar storyline to MongoDB -- the database and the company -- but their technology stack and solutions it provides and the TAM it has are a bit tougher to understand. So today, we dive deep into Elastic, and its suite of technologies that underpin its appeal to customers, and its new product lines spinning from that core.
How do I know the company's products so well? Besides being a software developer that works with a lot of databases and data feeds, I have worked with Elasticsearch (not the full ELK stack) for the past 4 years, using it as a vital piece of my architecture. More recently, I have run some parts of my stack within the AWS environment the past year (more for the data storage resources than compute). I'm about to try using managed Elasticsearch in AWS, and, besides it being a data store within my stack, I'm also about to start using ELK for APM and monitoring of my stack. [No brainer that I should have implemented long ago, it's just I don't have the time; too many other interesting projects (around data streaming) to do!]
Warning: There is a lot to like in Elastic, in ways that excite me beyond what MDB is doing. But for that, you'll need to do a lot of reading below to get to the Final Thoughts. But don't just jump there... I recommend the middle bits too! Dammit, don't miss the haiku! My last deep dive into the tech behind a company was Okta -- and this deep dive is even longer. For one, I know the company way better. For two, it was worth diving in deeper into their strengths.
Elastic Overview
Elastic is known for its suite of products it calls the Elastic Stack. (It was first called the "ELK" stack after the first 3 products, and it's still mostly called that.) After starting as a company focused on its Elasticsearch search database, Elastic shifted gears early on towards being a solution for specific use cases, when it acquired companies with complimentary tools, then integrated them into a platform around its core engine.
Their Getting Started docs describe the core well enough:
Elasticsearch is a highly scalable open-source full-text search and analytics engine. It allows you to store, search, and analyze big volumes of data quickly and in near real time. It is generally used as the underlying engine/technology that powers applications that have complex search features and requirements.
Elastic Stack is:
- Elasticsearch, the search and analytics database at the core.
- Logstash, the data processing and transformation pipeline, for data ingestion into Elasticsearch.
- Kibana, the visual interface over Elasticsearch, with data visualization dashboards and a cluster & data management interface.
- Beats, light-weight data shippers utilized for transmitting monitoring data from network and systems, and ingesting them into Elasticsearch.
- "Features" (formerly X-Pack), plug-ins that are modules for enhancing the capabilities of ELK stack, such as adding cluster monitoring, alerting, data security, reporting, machine learning (ML), and a visual presentation app called Canvas. [Not sure why Elastic decided to now blandly call it "Features". I guess they let the new marketing intern have a go at it.]
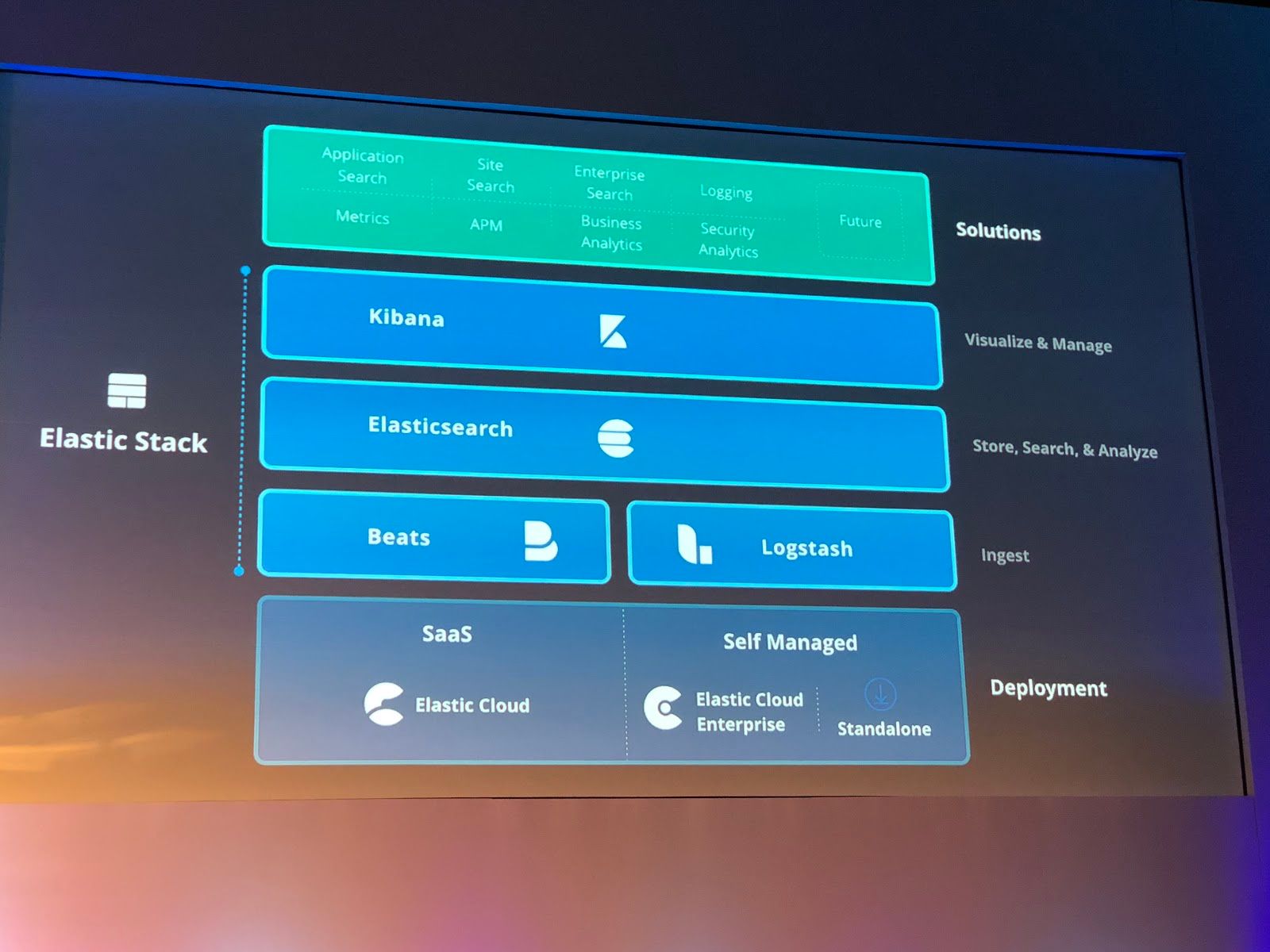
Elasticsearch (ES) is the open-source NoSQL database at the heart of the stack, which provides search and analytic capabilities over your data. It is built over the open-source Apache Lucene indexing engine (created by Doug Cutting, eventual creator of Hadoop), but with a focus on cluster capabilities to manage and search over ever-growing datasets. It is a distributed software, making the engine as powerful as the cluster hardware it is installed on, and the cluster can easily expand over time. A developer uses a REST API interface or native Java libraries to store JSON data, and can then search or analyze that data via the query interface. Bottom line - if you are slicing and dicing over large data (hundreds of Gb or more) or big data (hundreds of Tb or more) for search or analytical purposes, Elasticsearch is ideal. It is popular, having 40k stars on Github and a high DB Engine rating (#7 overall and #1 for search). There are a few competitors in the open-source space... but as I discuss later in detail, the real competition to Elastic is elsewhere.
Alternate open-source search-based databases on the market are:
- Apache Solr, which is also based on Lucene. But it pales in comparison, having only 2.5k vs 40k stars for ES on Github as a sign of its popularity, and #16 on DB Engine ratings. It has very little of the surrounding ecosystem of tools that ES has. It emerged out of CNET, and later was merged with the Apache Lucene project itself. An company, Lucidworks, was created to support it, and they created their own enterprise edition of Solr called Fusion. [Contrary to anecdotal commentary on a recent post, this is not a company I would look to as supplanting Elastic in any way. But sure, let's say, officially, it is a direct competitor.]
- Apache Druid, a OLAP/BI analytics engine, which has 8k stars on Github. It's also a clustered data engine but is a lot more convoluted & complex to run. It came out of work done at Metamarkets, an marketing analytical SaaS company, and now has an enterprise company, Imply, supporting it. Druid is a columnar store, which means it tracks field data together, not as separate rows. This allows for advanced analytics. [I'd keep my eye on it, but ultimately it isn't gaining much momentum, likely due to the complexity of the cluster setup required.]
Kibana is the visual interface over Elasticsearch. At it's core, it's a visualization dashboard app that allows you to rapidly graph ad-hoc queries against your ES data, and create persistent visualization dashboards. It is pretty similar to another open-source visualization dashboard, Grafana, but is more closely tied to having ES as the underlying database, and, unlike Grafana, provides a mgmt interface over your ES cluster and its data. One great feature of Kibana is its "out-of-the-box" dashboards for specific server apps you are monitoring with Beats, that are curated by Elastic; as you hook up monitoring over your server apps (say a PostgreSQL database or an Nginx web server) with FileBeat and MetricBeat, you can use Kibana's dashboard templates honed for that specific application as a starting point, then customize it from there as desired. It has plug-in modules for time-series data visualization (Timelion), geo-spacial visualization over maps (Elastic Maps), and exposes dashboards over many of the X-Pack features like ML anomaly detection and APM monitoring.
Logstash is the ingestion piece, that allows for continuously reading in logs from various servers, transforming log entries into JSON objects and ingest them into ES. It has a rich system of data pipeline steps, where you can convert, enrich, filter and transform log data prior to ingestion.
Beats is a collection of light-weight "data shippers", each specific to collecting a type of data feed from remote servers or devices. This includes a log file shipper, metric shipper, network traffic monitoring and more. They are installed as system agents on your servers, which allow you to continuously collect data and ingest it into ES. Each beat comes with a wide variety of server apps it can work with out-of-the-box. On the opposite side in Kibana, it includes sample dashboards for each server app, curated by Elastic staff, that are honed to that app's metrics or logs.
The Beat flavors are:
- Filebeat for log or data files monitoring. It can tie into logs from server apps for log monitoring of your systems (MongoDB, PostgreSQL, MySQL, Nginx, Redis, Kafka, etc), and can do container log monitoring of Docker and Kubernetes.
- Metricbeat for real-time server metric monitoring, including system-level metrics (like cpu/disk/memory usage, or temperature readings) and server app metrics (MongoDB, PostgreSQL, MySQL, Nginx, Redis, Kafka, etc). It can perform real-time metric monitoring of your stack, and can do container metric monitoring of Docker and Kubernetes.
- Packetbeat for real-time network traffic & latency monitoring.
- Auditbeat/Winlogbeat for system-level auditing of Linux/Windows systems.
- Heartbeat for system uptime monitoring. Simpler/lighter uptime check than Metricbeat.
- Functionbeat for real-time serverless function monitoring (i.e. monitor your AWS Lambda function).
Features/X-Pack is a system of modules within the Elastic Stack to enhance the platform and help apply Elastic Stack to specific use cases. [I included link to marketing materials on the high impact ones. It's worth reviewing and seeing what kinds of visualizations are possible in them.]
- Monitoring for cluster & server app monitoring.
- Security for role-based access security over your data, down to document/field level.
- Alerting for cluster alerting & data monitoring via queries (get notifications on spotted errors).
- Reporting to generate, schedule, email reports.
- Canvas for interactive presentation visualizations from real-time queries.
- SQL to allow for ES querying via well-known SQL data querying language.
- Hadoop Connector to directly connect Elasticsearch directly to Hadoop for querying.
- Graph tool to explore relationships in your data (use cases: fraud detection, security analysis, user recommendations).
- ML for performing machine learning over your data and visualizing results (use cases: detect anomalies, isolate patterns, pinpoint causes, demand forecasting).
- APM Server (server-side app) for collecting APM metrics from your application code and saving it to ES.
With X-Pack, they used to all be Premium and only come with Enterprise Support tiers. However, back in April 2018 they changed their licensing. (MDB soon followed suit with their licensing change in October 2018.) This made a new pricing tier, Basic, that includes several modules that are now free. [More later on the licensing changes.]
Elastic has a lot of out-of-the-box solutions here for monitoring. You can plus in Filebeat to pull PostgreSQL. you can tie in APM into your application code, and there is a Kibana dashboard templates, curated for each server-side application, that you can start with to visualization those metrics and logs.
There are a few other ancillary services and products that Elastic has:
- Elastic Map Service to provide high-quality global and regional maps, with which you can overlay geospatial data in Kibana.
- Elastic Common Schema is an effort from Elastic to try to unify data schemas for common activities (logs, metrics, APM, networking data).It is an attempt to unify how to look and visualize and analysis like data from different sources (say, Cisco's firewall logs vs Fortinet's). Elastic is hoping to convert various data sources into a single common schema, which allows you to simplify the search, analysis and visualization activities you do on that data.
Getting Support
There are 4 tiers of support subscriptions.
- Open-Source (free) - includes ELK, most of Beats, limited Elastic Map Service
- Basic (free) - includes free X-pack modules (monitoring, SQL), and all of Beats and Elastic Map Service
- Gold - provides biz hours support, plus includes rest of X-Pack (w/ only the basic Security, and no ML), and Elastic Monitoring service
- Platinum - includes 24/7/365 support, plus all the above, adding full ML & full Security modules (SSO, ACLs down to document field, encryption at rest), cross-cluster replication.
I griped above about how X-Pack is now just referred to as "Elastic Stack Features", a kind of bland label instead of a product name. But after reviewing that pricing chart showing features that turn on and off per tier, they are clearly blending in these modular features now; they are now directly embedded within their product lines, like ML and Security both being heavily integrated across all of Elastic Stack. I think they are losing "X-Pack" because they don't want to think of them as separate plug-ins -- they are integrated modules, and that has only expanded across other parts of the Stack. Kibana, Beats and Logstash have internal modules that turn off and on. This is why the open-source purists are upset -- there are proprietary modules embedded inside open-source packages. But, it seems Elastic is pretty clear about what is and is not included in each tier. Though its a bit too detailed, perhaps - they need some higher level overview of what modules are turning on and off. Support levels are spelled out clearly. You have to go Platinum tier for ML capabilities and advanced Security features. For Gold & Platinum support levels, they offer custom training and consulting services for additional fees.
Hosting
As for hosting their stack, a customer has the typical options plus an extra one for running your own on-premise cloud:
- Host it themselves (self-managed, self-hosted), either on-premise or in the cloud (on EC2 or Docker instances). It's a complicated software to configure, but obviously doable. I've used it entirely for free thus far -- but elsewhere in my company they just bought enterprise support to use Elastic Stack for monitoring infrastructure (system logs [Filebeat] and metric collection [Metricbeat]).
- Elastic Cloud is their hosting service, where Elastic can host and manage Elastic Stack clusters for you in your cloud-provider of choice. Elastic Cloud service came from their acquisition of Found in early 2015. MDB's Atlas probably took cues from Elastic and others, and released in mid-2016. Managed vendor-neutral cloud hosting is the big reason these companies are growing revenue so strongly.
- Elastic has a 3rd option, Elastic Cloud Enterprise, which allows a company to deploy Elastic Cloud onto its own infrastructure via Docker, and use it as an internal, on-premise cloud where they can create and manage multiple Elastic Stack clusters.
Comparison to MongoDB (MDB)
While they are similar, it is important to note upfront that MongoDB is a general-purpose document store with a wide set of use cases, while Elasticsearch is a specific-to-purpose document store with a narrower set of use cases -- having much better search & analytical capabilities, and well as more flexible scaling.
There are many similarities between them ...
- Both are focused around a core open-source NoSQL document store, accessed via JSON-based REST APIs or native libraries. Both engines are cluster-able and can be horizontally scaled easily (by adding more nodes to the cluster). Both data engines are built on replicated shards, which enable high availability (HA), resiliency and scale.
- Both founders created companies around that open-source database engine that provided enterprise support and continued adding features, tools and eventually platforms around their core database. Both then expanded to create managed vendor-neutral cloud hosting of their data engine (MongoDB Atlas vs Elastic Cloud). Bonus for both over the cloud vendors: the fact that the authors and maintainers of these data engines are the ones best suited to running a managed instance. Put the experts in charge!
- Both provide platforms containing tools around that core database. Both have apps for managing the cluster, data exploration and visualization. When you buy MDB Enterprise Advanced subscription, beyond enterprise support you get to use their mgmt interface app (Ops Manager or Cloud Manager) for monitoring and backup, advanced modules for security & analytics, data visualization tool (Compass), and also get a commercial license to embed Mongo in your released product. When you buy an Elastic Stack Enterprise subscription, you get enterprise support as well as all premium X-Pack plugins for security, auditing, and machine learning (ML).
Yet, some major differences ...
- MDB has wide variety of use cases, as an all-purpose document store. Elastic has narrower set of more specific use cases - however it is expanding. If you manage a collection of data objects that has infrequent search or analytics needs, you pick MDB. For data that you need to slice and dice continually with queries to search and analyze it, you pick Elastic. And for data that is "ever growing", you pick Elastic.
- Given this more limited set of use cases, Elastic has had to fight harder. They have mostly expanded their product line by acquisition, adding tools and services that helped build their core database into a platform. MDB is building its platform itself, and IMHO is subsequently moving way slower.
- Both have an "Open Source" focus, and both try to address having competitors use their software against them. However, they have different approaches on how to address their open-source licensing to combat competition. MDB is trying to prevent cloud-providers from running a hosted cloud service using MongoDB (making them direct competition against their own Atlas service), by changing the licensing on their core database (making the OSS purists angry). Elastic is keeping the core database fully open-source (Apache 2.0 license), but is changing the licensing and open-source strategy of their bundled 'mostly free' X-Pack modules (also making the OSS purists angry, but this time its about the fact it's bundled in ELK). Elastic seems content to let cloud-vendors be competitors to Elastic Cloud, and letting their feature-rich additional modules be the differentiator.
- Speaking of ecosystem, Elastic released a set of plug-ins for the ELK stack in 2016 that they called X-Pack, for non-core features like monitoring, security, alerting, and reporting. They started with all plug-ins being Premium (enterprise license required), but now source code is publicly available, but not open-source, and these modules are now free-to-use in their Basic tier. A subset of them are still premium and require an enterprise subscription. The Elastic Stack releases are bundling the open-source and Elastic licensed modules together. (Yes, this may cause some licensing confusion.)
- While both MDB and Elastic have a managed cloud-hosting service plus provide users support for their self-hosted & self-managed databases, Elastic has a 3rd option - Elastic Cloud Enterprise (also from the Found acquisition). It allows their Elastic Cloud product to be installed on your on-premise infrastructure, so you can easily manage multiple ELK clusters on an internal cloud.
- Elastic isn't building a cloud side and a on-prem side to their platform like MDB is. It's all Elastic Stack in the Elastic Cloud, just hosted at whatever cloud provider the customer desires, and managed by the finest experts one could find -- thems that wrote it! There isn't tooling appearing in Elastic Cloud that isn't in core platform, unlike MDB with their Stitch serverless platform. However, their releases must bundle the proprietary modules side-by-side with the open-source products.
Best of all, Elastic is making exciting moves that are moving their company beyond being a tool provider. There is something afoot! [More on that soon under TAM section. Keep reading! But I'll give you a hint, it rhymes with "class".]
Strengths, in Haiku
With Better Search
An ever expanding TAM
To Infinity
- muji, TMF Poet Society, May 2019
... More Insights with a Better Search and Analytics Engine
Elasticsearch provides much, much richer SEARCH and ANALYTICS capabilities compared to document stores like MDB. Elasticsearch has a more of a learning curve (having a more convoluted API format, and more complexity in setup and usage), but that is for a reason -- it does a LOT more.
Relational database has an engine that can host separate databases, each having a set of tables containing rows, columns (fields), indexes. Think of it as an Excel workbook (database), having multiple workbooks (tables) full of rows and columns. Each row has a statically defined set of fields in columns, and typically has a unique identifier (primary key) used for retrieving that row. Each table has indexes, that allow for faster filtering & sorting capabilities across its rows in predefined ways. "Relational" is about how data is able to join together - in Excel, it would mean the workbooks would be to reference the rows in other workbooks (say, tracking a relationship between a workbook data by storing an id of a row in a lookup tables in the table referencing it). You query, insert, update and delete data via a "structured query language" (SQL), which is standardized across the industry. You can calculate statistics via aggregations in an ad-hoc way via SQL (GROUP BY, SUM, AVG, MIN, MAX, nested sub-selects, joins, etc).
MongoDB is an engine that can host multiple databases that contains one or more collections (tables) of like documents (rows), each with a set of fields (columns) that can be varied. Each individual document (row) can be looked up via its assigned uuid (primary key). It can also have pre-determined indexes of specific fields, for faster searching. Being NoSQL, there aren't really "relations" (joins) unless they are manually looked up in a followup query, or embedded as a sub-object in the document itself. (v3.2 did finally add a simple join mechanism that allows retrieving a child document from a separate collection.) You can calculate aggregations via an agg pipeline, which is a set of instructions to filter and group rows then calculate statistics over each group.
Elasticsearch is an engine that does ALL those same things MongoDB does. It is a cluster (database) that hosts multiple indexes (tables). Each index has multiple documents (rows) comprised of fields (columns) that can be varied. Each document has an assigned uuid (primary key) to retrieve that document. From there, however, the search, aggregation, and analytic capabilities are greatly improved in Elasticsearch's indexes over MongoDB's collection indexes. It too has an aggregation pipeline capability, where you can nest logic to group and calculate over results. However, it's analytical capabilities are much greater than standard NoSQL data stores.
Why is the search so much better? It's built on top of Apache Lucene, an indexing system that allows for quick lookups. Lucene started as a full-text search engine, but has greatly improved over the years in handling numerical indexes as well (which include timestamp and geo-location data). In particular, the numerical and geospatial indexing in Lucene has greatly improved over the past 3 years - helped by Elastic developers, who are major comitters to the Apache Lucene project.
Where Elasticsearch really starts to shine is in AD-HOC filtering and lookups. When you set up traditional indexes in a relational database, everything must be rigidly defined. MongoDB loosens up that rigidity a bit, but still requires pre-defining fields in indexes. Elasticsearch can act as ONE GIANT INDEX over your data that doesn't require pre-defining how you are going to look at and search on that data. Data can be any combination of structured (rigid collection of objects with schema defining each property) or unstructured (loose collection of objects with varied properties).
For analytics, Elasticsearch's aggregation capabilities are incredibly flexible, and allows for very custom nested aggregations. Aggregations can be done over search queries, to bucket the results into groups based on a criteria (repeatedly, if desired, in a nested hierarchy). You can either get the raw data or then extract statistics about each resulting aggregated group. For example, you can group results by year & month, then by city, then aggregate statistics for each resulting nested sub-group (like 2019-01, Denver). Time-series based data can be grouped into smaller time slices (say, calculate a rolling 5 minute avg of a metric over a month span). Geo-locations (long/lat) can be grouped into shapes and regions.
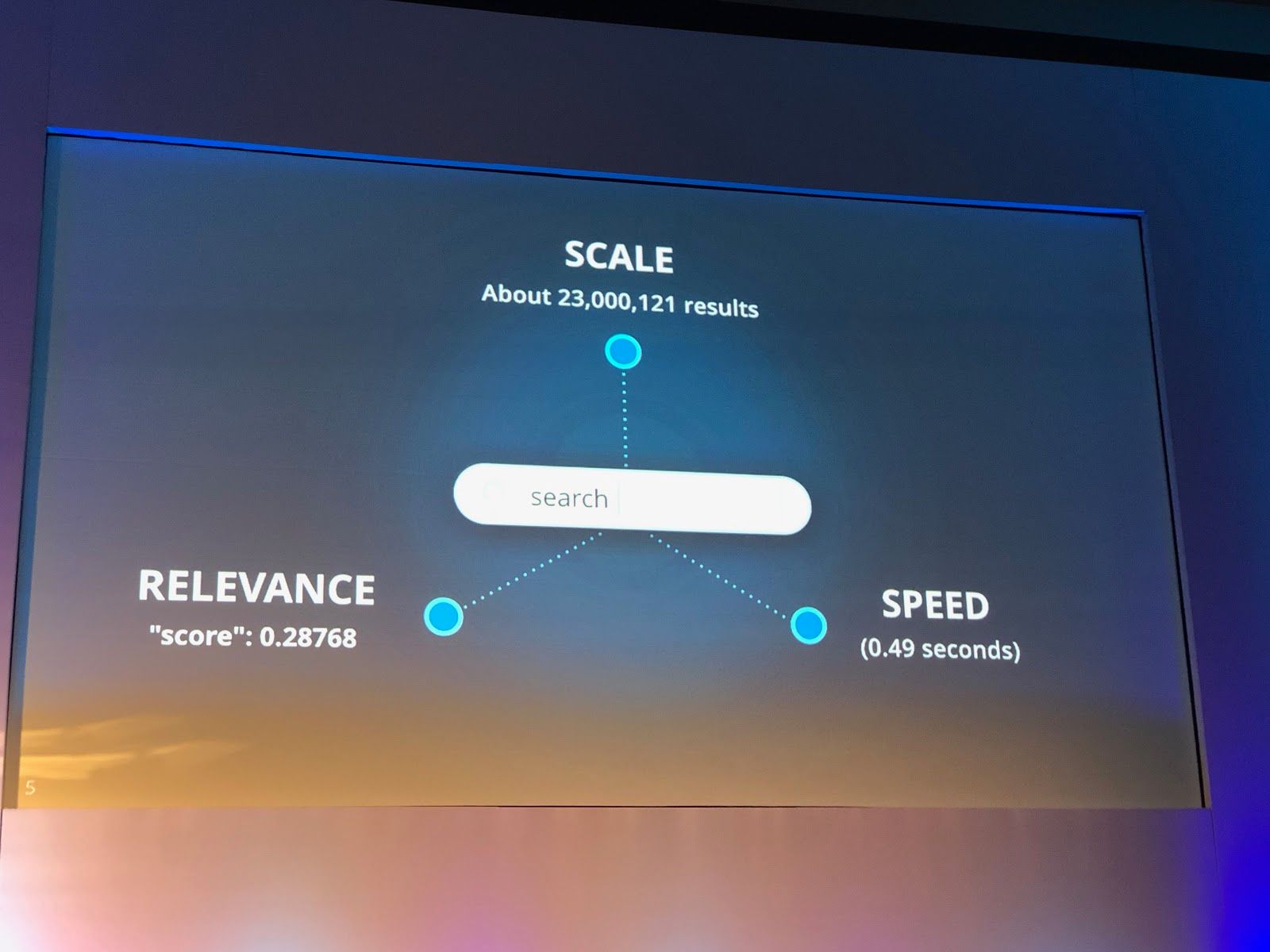
Elasticsearch does full-text searching through a scoring/ranking system. It exposes a wide variety of search methods over full text (word proximity, text variation matching, top X ranking, pattern matching, fuzzy text matching, misspellings, etc). The other search type is structured searching, exact matching or ranges on text or numerics. This includes many numeric use cases, for indexing and sorting and grouping numbers, timestamps, IPs or geo-locations. Numerics really open up the use cases - searching and analyzing time-series data (ie sensor data) and geospatial datasets (ie sensor locations) really shine within Elasticsearch, where grouping and aggregating by time period or by geo-location is handled incredibly fast. It is hands down better than relational database indexes over columns, and the improvement widens further as the size of the data grows.
As data is imported into Elasticsearch and being indexed, it can be set up to go through different text analyzers & tokenizers to split an incoming document into relevant search terms. This allows you to customize how the search internals work based on how you plan to use your data. It supports structured or unstructured data, and you can provide the structured layout (schema) as desired. You can also embed child data within a parent document and search through that.
Then you want to query the data, as you now have the ability to comb through your massive dataset and find the rows you want quickly (filtering) as well as ordering the data as desired (sorting).
Multiple indexes and aliases
One big strength of Elasticsearch is the ability to search over multiple indexes in a single query, including the use of wildcard. This allows you sub-divide your indexes into natural ways you plan on querying or managing it. For example, you can create a new index per month for the same core dataset. You can then query over any number of months (an index per) as desired. For ease of crafting queries, you can set up aliases that cover multiple indexes, so you only reference one alias instead of multiple indexes each time. Aliases can keep your query static over time, such as using an alias for trailing 12 months that you shift each turn of the month.
Filters
First thing you set up in your query is what is the overall data you want to view. You can filter by a timespan, or by US State, or however you want to slice and dice the data. Once you isolated that data, you can expand that filtered query with additional filters (nested filters), sort it, or then add aggregations.
There are 2 overall modes of searching:
Full-text searches attempt to match the most relevant documents to your filter. It can use relevance (rank) and token analysis, combined with boolean logic ops (like must, must_not, should), in order to generate a ranking score per document. You can create +/- score adjustments based on criteria, called "boosts", to better hone your results (ie searching on "tests", but not wanting "unit tests", so you demote it).
Structured searches make a boolean determination (a yea or a nea) per document. It allows matching against exact text (categories, tags, names, ids) or numerics (number, dates, times, geospatial locations). You can search by match, ranges, or use logic operations.
There are multiple types of queries over those 2 modes.
- Full-text queries - ranked full-text scoring (match, match phrase, multi-match, synonyms, stemming, bigram matching, phrase matching, fuzzy matching) and span matches (word proximity, order of words)
- Term queries - yea/nea text matches (exact terms, ranges, wildcards, and regex) like searching over tags, usernames, locations, etc
- Numeric queries - ranges matches against numeric operations (greater than, less than, between) including over dates, timestamps, geospatial and IPs
- Geo queries - geospatial location matches (within distance of a point, or within one or multiple bounding shapes)
- Join queries - search nested child rows in document
Beyond the large number of filters available in Elasticsearch, if you have highly specialized needs, you can use a custom script to create your own.
Aggregations
After performing a search (filtered query) you can also specify one or more aggregations, to group the search results into buckets or generate statistics. The power of aggregations is that they can be nested. For example, bucketing search results on state, then bucketing those results (per state) on city, then aggregating count on each group (per state & city).
Bucketing - Group data on criteria based on field(s)
- Terms, Significant Terms, Significant Text, Filter, Adj Matrix, Histogram, Date Histogram, Auto-interval Date Histogram, Composite, Diversified Sampler, Global, IP Range, Missing, Nested, Parent, Children, Range, Reverse Nested, Sampler, Diversified Sampler.
Metrics - Compute analytical stats over group
- Metric aggs: Top Hits (relevance), Stats, Extended Stats, Count, Cardinality, Avg, Weighted Avg, Min, Max, Sum, Percentiles, Percentile Ranks, Median Abs Deviation (variability), Scripted Metric, Geo Bounds, Geo Centroid
- Matrix - Group multiple fields into matrix
- Pipeline - Aggregate results from other aggregations
Filtered and aggregated queries open the door to all kinds of search & analytical capabilities.
... In a Complete Ecosystem that is Expanding TAM
One huge difference in their business strategies is that Elastic hasn't been afraid to acquire its tooling. Kibana and Logstash were both acquired in 2012, then in 2015 was Packetbeat the foundation of Beats. Found was acquired in 2015 to integrate as Elastic Cloud and Elastic Cloud Enterprise.
MongoDB is catching up here in terms of ecosystem. In 2015-2016 they added Compass (data exploration and mgmt console) and backup & mgmt tool Ops Manager, but it took until v4 release mid-2018 for them to add Charts (viz dashboards), and to begin expanding their tooling into a cloud platform with Stitch. MDB made Atlas themselves in 2016, then later acquired mLab in 2018 to acquire their customers and migrate them to Atlas.
MDB has created a great company, and has created many new tools, but wasn't always focused on creating a unified platform. Elastic has large number of bolt-on acquisitions, where it acquired all the pieces and parts of its ecosystem. This has allowed it to move much faster than MDB; but then again, it needs to, given its more limited use cases.
Licensing changes
Let's take a minute to talk about all the open-source licensing changes that have occured in these enterprise companies built around a specific open-source database. The eloquent Jay Kreps, CEO of Confluent (company around Apache Kafka), put the history of these types of companies very clear in his blog post about Confluent's licensing changes around Apache Kafka. https://www.confluent.io/blog/license-changes-confluent-platform
“The major cloud providers (Amazon, Microsoft, Alibaba, and Google) all differ in how they approach open source. Some of these companies partner with the open source companies that offer hosted versions of their system as a service. Others take the open source code, bake it into the cloud offering, and put all their own investments into differentiated proprietary offerings. The point is not to moralize about this behavior, these companies are simply following their commercial interests and acting within the bounds of what the license of the software allows.
As a company, one solution we could pursue would be for us to build more proprietary software and pull back from our open source investments. But we think the right way to build fundamental infrastructure layers is with open code. As workloads move to the cloud we need a mechanism for preserving that freedom while also enabling a cycle of investment, and this is our motivation for the licensing change.”
There are two strategies these "open-source" enterprise companies are employing:
- Attack on the edges, by differentiating on the surrounding modules/tools in the platform. (Elastic)
- Attack at the core, by preventing others from direct cloud-hosting. (MongoDB, Redis, Confluent)
MDB is not leading the charge here on the cloud front, either with cloud hosting nor with fighting the cloud providers. Elastic bought Found in 2015 to make Elastic Cloud; a year and a half later, MDB released Atlas in mid-2016. Elastic made licensing changes in March 2018. Redis next followed suit in August 2018, but with a different strategy; they instead changed the OSS license on the OSS core, to avoid cloud-providers hosting it. MDB followed Redis's path in October 2018, as did the aforementioned Confluent. Redis then later changed their licensing AGAIN, in March 2019. Which is the better or worse strategy here? Does MDB have to follow Redis again and change the license a second time, given the OSS community backlash? Elastic doesn't seem to mind the backlash, but they have a different strategy, and their core remains open-source (however still tightly controlled by them as sole contributors). [As we'll get in to soon enough, I pontificate later as to how their strategy completely enables a whole new front in Elastic's new product lines.]
Acquisitions
Elastic's acquisitions that added tools or SaaS services in their ecosystem:
- Kibana (2012) - Visualization dashboard app, that worked over Elasticsearch. Still here as Kibana, but its been greatly expanded with modules (Timelion APM, ML, etc) and now cluster mgmt interfaces (about time).
- Logstash (2012) - Log collection app that converts log files into data objects, and imports them into Elasticsearch.
- Found (2015) - A cloud-neutral managed Elastic Stack hosting SaaS service. Now is same named Elastic Cloud service and also the Elastic Cloud Enterprise "on-premise cloud" version.
- Packetbeat (2015) - real-time network packet analytics library, built ELK stack, to monitor distributed systems. Now is Beats product.
- Prelect (2016) - predictive behavioral analytics firm, focused on cybersecurity, fraud detection, and IT operations analytics. Now likely drives ML modules in Kibana.
- OpBeat (2017) - APM system for Javascript apps. Now an APM module in Kibana and the APM Server app.
- Swiftype (2017) - Startup providing hosted SaaS search service for enterprises to easily add search capabilities to their website or app. Directly competes against Google Search Appliance [RIP] and it's replacement, Google Enterprise Search SaaS service, as well as Google Site Search [RIP] and it's replacement, Google Custom Search Engine. Elastic rebranded these offerings as Elastic App Search and Elastic Site Search SaaS services, and then follows it with another - the Elastic Enterprise Search SaaS service just announced. This competes against the Google Cloud Search service, which recently expanded from searching G-Suite to include other 3rd party SaaS tools.
- Insight.io (2018) - Startup with a developer-focused SaaS tool for creating a search interface over your source code. Supports a wide variety of modern programming. It's not just full-text search over the code, it has semantic understanding of the code, so provides intelligence over your code base. Supports code cross-referencing, class hierarchy, functional understanding of logic & structure. [Wow! I could use this!] This seems an essential service for software development shops, allowing them to tie "code intelligence" over their APM, logging and infrastructure monitoring that Elastic Stack already excels at. I haven't seen any particular Elastic-branded module or service appear yet from this - but sounds like its something that would be kept as a SaaS service. So in the same space that Atlassian is in.
Let's review those acquisitions above -- Bolt on, bolt on, bolt on, bolt on... check check check check. I am way more impressed with Elastic than MDB here. Very natural expansion of product line and TAM as they consume apps and services around or, even more excitingly, built UPON Elasticsearch. Kind of like the Borg. Or Square, actually (Square for Restaurants, built upon Square platform and ecosystem of products.).
MDB is trying to move into the same ecosystem - enterprise plugins with a premium cost, mgmt interfaces, and (finally) a viz dashboard and improving analytical capabilities. But they are moving way slower than Elastic, and are never going to catch up to start stealing business from Elastic for search & analytical use cases. I think they are playing it safe & conservative, as they already have a wide set of use cases. Any collection of data in a modern web or mobile app can use MongoDB. You don't built a financial transaction service upon it, perhaps, but most everything else, surely!
As I said in my prior "MDB Goes Mobile" post: “MDB just had an acquisition, their third. Their prior one, mLab in Oct 2018, cost $68M, and allowed them to convert customers from an Atlas competitor (managed cloud-hosted MongoDB instances) into Atlas customers. While that last acquisition was a customer and team acquire, they just bought Realm for $39M to acquire product lines that help them jump start their new mobile initiatives.”
MDB had an acquisition to buy customers (mLab). They already had their own mobile database product and had a mobile sync service in beta -- yet they NOW decide, after that development effort, to purchase Realm as a bolt-on (and, I'm guessing, scrapping their efforts thus far towards mobile and sync). I can't tell why MDB is moving so slow - they keep building everything themselves! Charts, their new SaaS visualization dashboard service, was new in v4 released a year ago... yet is still in beta nearly a year later. Not impressive! They could have easily bolted on a viz dashboard long ago, instead they built it. (Taking, what, maybe a year, maybe more, to develop? Make that two... it's been 11 months since release and still is marked BETA.) And, a step farther, I don't see MDB making enterprise SaaS tooling anytime soon, either.
Elastic on the other hand, is expanding their platform to help them find more and more appropriate use cases for Elastic Stack ... OR ... to find new successful SaaS services built on top of Elasticsearch. Either way, they expand use cases and expand the potential TAM. There is nothing stopping them from making a competing services to Splunk or New Relic -- but for now they are focused more on enabling others to do that.
Or are they? Look where Elastic is starting to acquire or create new business-focused SaaS services. Swiftype, a hosted search service acquired in late 2017, is the incubator of these impressive new business-facing SaaS services. Elastic is now "eating its own dog food", as we say in the software biz (aka consuming its own services), by creating SaaS tools built on top of itself! Elastic now has new SaaS services, based on Elastic Stack under the hood. I linked to both branding pages. Elastic has renamed them, but seems to be leaving Swiftype segment to its own marketing - perhaps for existing customers and word-of-mouth.
Elastic App Search Service
https://swiftype.com/app-search
https://www.elastic.co/solutions/app-search
SaaS search service built over Elastic Stack. Allows companies to feed in data from a variety of sources (like their own databases), either on-premise or in the cloud, in order to allow search capabilities within their app over that data. [Someone tell The Motley Fool, that right there is their new board search tool!]
Pricing:
- $49/mo Standard - basic searches & analytics, 7d history, unlimited users (50k doc limit w/ +$25/25k add'l, 500k request limit w/ +$5/50k add'l, +$25 each add'l engine)
- $199/mo Pro - advanced searches & analytics, multi-lingual, 6mo history, cross-domain (100k doc limit w/ +$20/25k add'l, 1M request limit +$5k/50k add'l, $100 each add'l engine)
- $??/mo Premium - dedicated hardware & support, SLA
Elastic Site Search Service
https://swiftype.com/site-search
https://www.elastic.co/solutions/site-search
SaaS search service to add a site search capabilities. Site indexing. Provides search bar with autocomplete. Can leverage the power of Elasticsearch for text searches (spell correction, similar word matching, synonyms, phrase matching). Great for e-commerce, knowledge bases, cust support, media content.
Elastic is saying this is a replacement for the end-of-lifing Google Site Search service. They highlight Twilio using it on their API docs.
Pricing:
- $79/mo Standard - crawl up to once/day, multi-lingual (5k doc limit w/ +$25/5k add'l, 50k request limit, w/ +$25/50k add'l, +$50 each add'l engine)
- $199/mo Pro - crawl up to once/hr, PDF/DOCX indexing, cross-domain, analytics (10k doc limit w/ +$25/5k add'l, 100k request limit +$25k/50k add'l, $$100 each add'l engine, +$100 each add'l domain)
- $??/mo Premium - dedicated hardware & support, SLA
Elastic Enterprise Search
https://swiftype.com/enterprise-search
https://www.elastic.co/solutions/enterprise-search
New beta service JUST ANNOUNCED THIS WEEK (no pricing yet), that again emanated from the Swiftype team. SaaS search service that provides enterprises search over all of their SaaS team tools (Salesforce, Github, Dropbox and Google Drive content, Slack, Zendesk, etc). Maintains user security (user searches only their content they can view). Wide variety of SaaS services supported, and you can +/- boost the search priority of each. If your tool isn't supported, they have the ability to add custom connectors. Fantastic expansion of the Swiftype platform. Their marketing maintains a great balance - paraphrasing but basically said "we can do this as a SaaS service for you, or build it yourself with Elastic Stack".
As we discovered in the blog post announcing it, you can run this on Elastic Stack yourself with Platinum tier enterprise license. Great way to up sell the licensing -- ML is another feature you need Platinum tier for. [Given it ties into Github, I wonder if they will tie in the "code intelligence" platform from Insight.io, or if that stays its own thing.]
All of these new services have enormous potential as enterprise SaaS apps in their own right. Perhaps not as sticky as a service like Okta, as it competes with Google on all of them. But it seems like just the tip of the iceberg as far as what Elastic could do here, in terms of search or analytic enterprise SaaS tools.
... That Can Scale Up to Infinity, and Beyond
We live in a technological world where datasets are ever-growing, as you pull in time-series data feeds from monitoring IoT sensors or infrastructure. In some cases, data can get stale and need to be discarded -- either archived, rolled up (summarized), or dropped. In other cases, especially around analytics, you want the entire dataset kept, as a "data lake" (a pool of all your raw data) containing all their internal knowledge and data. The cloud allows this, as you can scale up your compute/disk capacity as needed. [There is still a place for "data warehouses", which is more a pool of processed, filtered, or rolledup data, as opposed to raw data. One such up-and-comer is Snowflake, a cloud-hosted SaaS data warehouse service. I would definitely look closer at them if and when they are go public.] Regardless, any company's data needs are large and only going to continuously grow from here.
There are 2 ways to increase the capacity of a data engine. Vertical scaling is increasing a server's capabilities (giving it more disk, more RAM, increase network bandwidth). This is what you were limited to in the "olden days" of relational databases. However, if the data engine is capable of running as a cluster, comprised of one or more nodes (individual systems), you can horizontally scale, which is increasing the number of nodes in that cluster. Each additional node added to the cluster increases capacity and capabilities. Both MongoDB and Elasticsearch are clustered data engines that utilize replicated shards. (Though, to be clear, MongoDB can and typically is run standalone. Elasticsearch's setup for production is more like 3 nodes minimum. It was built on and for clustering from the start.)
Sharding (also called partitioning) is a way to split up a data set across a cluster, to allow you to 1) horizontally split your data, to be able to scale the performance and size of your cluster, and 2) allows you to distribute and parallelize operations across shards located across cluster nodes, to improve performance & throughput.
So, as an example, say your dataset has 5 shards, each containing 1/5 of your data. Those shards can be located across multiple nodes within your cluster, splitting up the work load and memory/disk use.
An important aspect that shards enable is shard replication. Each shard is a fraction of your dataset, and by replicating that portion of your dataset across nodes, you are able to 1) scale your querying capabilities (each node does searching and aggregating on shards it holds) and 2) prevent data loss by always having a replica to fall back to ("high availability"). As search queries come in, they can go to any node in the cluster that has a copy of that replicated (read-only) shard. A replication factor of 2 means each shard is replicated 2 additional times, to 2 different nodes than the one the primary shard resides on, so that a total of 3 copies of that shard always exist. That replication factor would triple the concurrent search requests that can occur simultaneously (splitting up the load between the shard replicas, and so between nodes of the cluster). It would also allow for up to 2 nodes to simultaneously fail without data loss occurring -- if a node goes down, a replicated shard on another node can turn into the primary shard (that new data is written to and that is cloned to the other replicas), and a new replica is created to take its place.
Scale (enabled by shards and replication) is everything when dealing with clustered data engines. The primary reason folks are moving off of SQL databases is the lack of horizontal scale capabilities in today's data driven world. Huge volumes of incoming data means your cluster needs a large ingest capability. Large data sets require a lot of disk and memory to handle it. Having a constant stream of continual queries means your cluster needs a large search capability and network bandwidth. Relational databases, unfortunately, have been really left in the dust here -- there are tricks you can play with read-only replication, but there are very few clustered SQL engines that can horizontally scale.
Taking clustering capabilities a step further, an "elastic" cluster is a "smart" cluster, one that knows when nodes are added and removed from the cluster, and is able to balance the data across all the available nodes. It gives "high availability" by being able to keep search and ingest continuously available during node outages, plus is able to balance shard data across new nodes as they are added. I think you can tell where Elasticsearch gets its name.
Unlike MongoDB, Elasticsearch also provides the ability to scale indexes (tables) internally. An individual "dataset" in Elasticsearch can and should be MORE THAN ONE INDEX. That gives developers a huge improvement in how they manage scale of individual indexes (tables) within the cluster, and to control how massive datasets are segmented for queries.
Within a given dataset, you can split indexes on a time frame (say, spawn a new index daily). You can specify multiple indexes per query, plus use wildcards in order to search over split indexes in a controlled way. As an example, you are splitting a base "system-metrics" index per day, tracked as a suffix on the base index name (e.g. 2018-01-01). 2018 data can easily be queried via a wildcard search to "system-metrics.2018-*", or just query the first quarter via "system-metrics.2018-01*,system-metrics.2018-02*,system-metrics.2018-03*" (which would search over 90 different indexes of the dataset, one for each day). You can also utilize aliases in Elasticsearch, that are mapped to a specific grouping of indexes. So that last examples above could be more easily referenced in the future as "system-metrics-2018q1".
A dataset could be set up as one giant index that contains all the data and you would use query filters to narrow the timespan of your search. However, splitting indexes across time period (or other factors) greatly enhance SCALE and MAINTENENCE of that index. This provides many ways developers can gain huge improvements in speed. In using honed queries that are optimized to your split strategy, you can greatly reduce how much data within that dataset to search through. So instead of searching over the one big "uber" index containing all the data over time (say, 20B documents), you can isolate your searches to query only the subset of the time period you need (say, the month of April, which is only 10M documents). As new data arrives, it can get split up into individual indexes per time period (known as Rolling Index strategy), so datasets can ever-grow (scale continuously) by creating new index once a new time period is reached as a split point. This greatly helps a user manage the lifecycle of their indexes, by allowing them tools to curate their data as it continues to stream in. And as data ages, you can trim stale or archived data very easily, by simply removing the specific split indexes covering the time periods you wish to trim (instead of the traditional SQL method, of having to do a filtered delete query). Or you can set latest data to be on the fast hardware and older, less reference indexes to slower systems (known as Hot/Warm architecture).
Add up all the scale capabilities, and Elasticsearch is a data engine capable of storing and querying a massive amount of data in "near real-time". It can handle a fire-hose of incoming data (indexing it in a shard quickly, then replicating it to other nodes) so as to make the incoming data immediately available to ad-hoc searches and aggregations.
This makes Elastic Stack very relevant for "Do-It-Yourself" style use cases like monitoring. You can make your own Splunk or New Relic, and greatly reduce your app and infrastructure monitoring costs. You can make your own security analytics platform,
Use Cases
Companies need a scalable database to handle search and analytics over a LOT of data, including ever-growing datasets like metrics. There are lots of reasons to integrate Elastic Stack into your infrastructure. Full-text search was just the start. Elastic Stack excels at search & analytics over:
- Full text data (ie articles, blog posts, tweets, comments)
- Terms data (ie tags, usernames, locations)
- System logs & real-time metrics (ie systems, network devices)
- Application logs & real-time metrics (ie server-side apps, databases, APIs, microservices)
- Security/Audit logs (ie firewall logs, app audit logs)
- Numerical data (ie financial tx analytics)
- Time-series data (ie metrics, events, devices, IoT sensors)
- Geospatial data (lat/long points, geo-regions, location beacons)
- IP data (network traffic, routing logs)
These data-types combine into multiple use cases they market against - but these are just the tip of the iceberg. Expect the use cases to continue to expand from here as they come up with more use case combinations as well as address more verticals specifically.
Infrastructure Monitoring
- Logs https://www.elastic.co/solutions/logging
- Metrics https://www.elastic.co/solutions/metrics
- Application Performance Monitoring (APM) https://www.elastic.co/solutions/apm
- Uptime https://www.elastic.co/solutions/uptime-monitoring
Elastic is really pushing a wide variety of time-series and geo-location based use cases around monitoring; IoT, sensor, app, network and infrastructure monitoring are all heavy use cases of Elastic Stack.
Elastic is really going after do-it-yourself infrastructure monitoring. There are 3 overlapping angles to using Elastic Stack for monitoring your infrastructure. 1) It can ingest and search over log files output from your systems and server apps, like syslog and database logs. 2) It can ingest and search over real-time metrics from your systems (like cpu/memory/disk/network usage) as well as your server applications. 3) Then it can utilize APM, where you ingest your app logs straight from the apps. It ties into your code directly via an APM library, available across a wide variety of software languages (Java, Javascript, Go, Python, Ruby). This becomes particularly needed if you have distributed code base or use microservices strategy, where you really need to monitoring the flow of communication and data between all your modules.
Same for networking and security monitoring. You can pull in logs from routers, firewalls and other networking equipment. Then use ML module to isolate anomolies, or view traffic hotspots on regional maps.
So Elastic Stack allows and organization to watch their own infrastructure, networks and app stacks. This enables companies to do-it-themselves, for a fraction of the long-term cost of Splunk, New Relic and Datadog.
Search services
- App Search
- Site Search
- Enterprise Search
It's a search engine at the core, so if you need search within your enterprise, on your web site, or within your web/mobile dapp, you are in the right place to Do-It-Yourself and embed Elasticsearch into your stack. Beyond that, Elastic is making some moves here with SaaS services that provide these search capabilities directly to enterprises, without the need to manage or host the Elastic Stack. (More on this later.)
Analytics
- Security Analytics https://www.elastic.co/solutions/security-analytics
- Audit
- Business Analytics
- Mapping https://www.elastic.co/solutions/maps
Once you have your data flowing into Elastic, you can leverage the analytics capabilities for security and audit purposes. Utilize the ML module, or pipe it into your own analytical package (Spark, Hadoop, AWS EMR).
You can use any kind of geospatial data in Elastic Search, to group together like locations, and create group into hotspots on maps. You can look at traffic flows between geo-shapes (cities, countries).
Competition to the data engine is MongoDB, Cassandra, and other scalable NoSQL stores. Developers may prefer and pick those. But the search and analytical capabilities of those pale in comparison to what Elasticsearch does.
Amazon is a competitor to hosting Elasticsearch. Unlike MDB, Elastic isn't combating it via licensing, but instead are combating it with their proprietary XPack plugins. To me, MDB and Elastic have different licensing battles for the same purpose - combat the cloud-vendor alternatives. MDB is trying to prevent them from using MongoDB altogether, while Elastic is trying to have differentiated features.
AWS comparison, from Elastic marketing, says they are missing:
- premium modules for ML, Security, Canvas
- free modules for alerting, monitoring, SQL, Canvas
- curated UI plugins for monitoring/APM
- Index curation & rollup features (Hot/Warm/Frozen indexes)
- Elastic Map Service
- Logstash/Beats management UI
I think the major competition is their competitors in their use cases. SaaS infrastructure monitoring companies like Splunk, New Relic, Datadog, and the like are losing customers tired of the high monthly charges, who can build it themselves on Elastic Stack for a fraction of the cost. ELK stack is for DO-IT-YOURSELFERS and those on a budget, compared to the using those SaaS tools with ever growing monthly expenses.
Final Takeaways
Elastic knew early that they needed a complete ecosystem. Kibana is a data visualization dashboard, but also provides the interface to manage the cluster and the data within. Logstash and Beats both enable using Elastic Stack without coding. Elastic has a major focus on ML over the data, for things like anomaly detection and thread detection. In comparison, MDB has been catching up on ecosystem tools like Charts, but has nothing around analytics or ML tied in.
Yes, MDB has a much wider use case. But for search and analytics, there is really no alternative to Elasticsearch outside the way-less-used Solr. The choice for a company is really, does a search engine apply to our use case? If so, you go Elastic Stack. So the question of competition is really if you use Elastic Cloud or AWS to host your managed cluster. MongoDB is solely used by software development companies. Elastic Stack can be used without code! That means that, unlike MongoDB, it's not just for software developer companies -- any company can benefit. IT departments are using it just as a standalone Elastic Stack, directly integrating monitoring capabilities without needing any custom development effort. Kibana is a very easy-to-use visualization dashboard tool. IT can install Beats onto infrastructure, and suddenly it is all feeding into your cluster for DO-IT-YOURSELF monitoring.
I have spoken about Elastic before, as I attended and wrote up their ElasticON developer conference last October. Their main focus at the conference was for 2 main customer use cases: use it to monitor everything (logging + metrics + APM), and use it to help secure your network & infrastructure by building a Security Event Information Management system (SEIM). I dove into more details about those use cases there. One highlight I focused on was how a company brought their SEIM system from Splunk to in-house, and they stressed costs going from "$$$$$" to "$$$". Simply put, companies with large infrastructure can save big bucks by taking a DO-IT-YOURSELF attitude with monitoring and security. Elastic directly competes with Splunk and New Relic here.
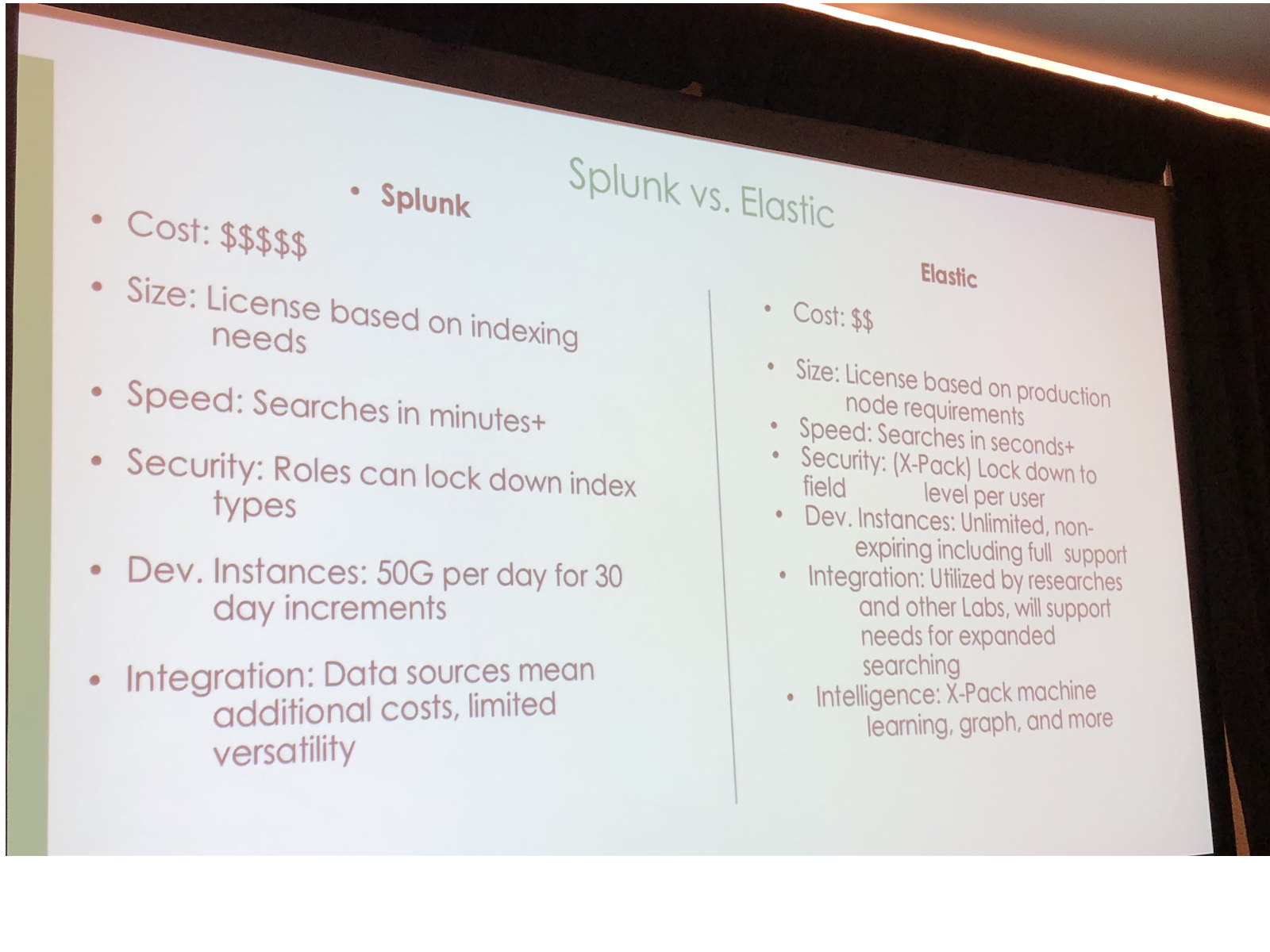
Elastic has a land-and-expand philosophy with customers, as they feel if they can get a new customer to use it for one use case, then they will find all their other use cases for it and start expanding their use from there. If on Elastic Cloud, managed hosting fees will likely increase over time. If on support, customers may rise up the pricing tiers as their dependency increases.
Elastic & MDB are similar companies with similar products doing similiar things. Their product lines are converging. They are NoSQL databases that compete, and have closely matching business product strategies (both heavily focused on cloud-neutral managed hosting). MongoDB is adding visualization dashboards and analytics. Elastic is adding better mgmt capabilities and widening its use cases. Both have tried to differentiate their managed cloud-hosting service from AWS's. At a minimum, both are the authors of the database, so are absolutely the best resource to host that database for you and help you with it. But beyond that, MDB offers Stitch and Canvas, and Elastic Cloud offers premium X-Pack features. AWS is starting to fill in the gaps with their "Open Distro for Elasticsearch", but they only cover a few of the basic X-Pack plugins so far (security and alerting). They aren't going to catch up to Elastic Stack's features like this. Elastic is more than happy to highlight what AWS Elasticsearch cannot offer in their marketing.
So the licensing battles are just a strategy difference on how to fend off competition from using their open-source core in a competing hosting services. MDB is fighting via their core licensing. Elastic is using their ecosystems plug-ins to differentiate their platform. Google and Microsoft are choosing to partner with Elastic for managed Elastic Stack hosting on their platform, instead of building a competing service. Amazon is fighting it to the point of branching their own "Open Distro of Elasticsearch" that doesn't include X-Pack plugins, instead has open-source security, alerting and SQL modules AWS has written. AWS doesn't typically contribute to open-source. They aren't doing this out the kindness of their heart - they cannot sell managed Elasticsearch clusters without these features being present. Expect Elastic to continue expanding X-Pack features to differentiate themselves. I can't believe AWS gets away with this competitive behavior (making a service that competes with your major AWS customer), but I guess AWS's behavior is par for the course for Amazon. When MDB changed their license, the press sold it as combating Asian cloud providers, but in reality the first front was AWS.
I am going to take it a step further -- "Open Distro of Elasticsearch" shows me that AWS cannot compete against Elastic Cloud with just the core Elasticsearch, as they had to find a way to use the X-Pack features but they couldn't (as that is prevented under "Elastic License" they are under). Different license game than MDB, but I think it's working just as well. AWS has to find another way to differentiate their service from Elastic Cloud (besides price -- yes, AWS is cheaper). I think they are starting to, as recently on AWS marketing is pushing AWS Kinesis as a data stream that can easily integrate directly into AWS Elasticsearch.
[Side bar to the whole "open-source database company doing cloud hosting" part: Confluent, maker of Apache Kafka, is one to watch for going forward. Kafka is a data streaming platform on a high-availability cluster. Not a database, per say, but damn close (more a persisted message queue). Disclaimer, I am a database developer that uses Kafka a lot. Confluent hosts Confluent Cloud managed hosting service, and so if & when it becomes public, I would consider its numbers and put it up with MDB and Elastic as an extremely sticky platform for software development companies. AWS runs a competing AWS Kinesis service, but now also runs its own AWS Managed Kafka service, as that platform has a lot of momentum.]
Elastic has a land-and-expand philosophy with customers, as they feel if they can get a new customer to use it for one use case, then they will find all their other use cases for it and start expanding their use from there. If on Elastic Cloud, managed hosting fees will likely increase over time. If on support, customers will likely rise up the tiers.
Creating a managed cloud-neutral hosting service over the core platform is clearly a big money maker for these open-source companies. That's the current big revenue growth coming in. But Elastic is adding the next wave of growth -- creating their own SaaS services for enterprises. This is the two fold nature of Elastic's acquisitional prowess. It first bolted on tool sets around it's core, to build an ecosystem around it. But the recent acquisitions are altogether different. In Swifttype and Insight.io, it found companies that built itself on Elasticsearch (as they are allowed to, by the permissive Apache 2.0 license!) and both have a SaaS search service for enterprises. Such a superb direction for Elastic; they can leverage their expertise! There may be risks in this direction, but I think this has already been addressed by Elastic -- the marketing is taking a great tack in saying you can use the SaaS Service or do it on Elastic Stack yourself. Elastic is also keeping Swiftype an independent division, but they aren't hiding it (they tie Elastic and Swiftype web sites together well). It's such a good idea -- find companies building on the Elastic Stack, and acquire ones that align with Elastic's vision and tooling. They can leverage all their knowledge about the core Elastic Stack platform it is built on, but focus these SaaS services toward highly-honed enterprise solutions around search and analytics.
Beyond even all that, the recent acquisition of Insight.io really has me intrigued. They have a developer-focused SaaS service that integrates into code tracking services (Github, Bitbucket) and provides intelligence and search capabilities over your code base. This puts them into same developer-focused SaaS market as Atlassian. Which, it so happens, is a market that has extreme cross-selling potential to their existing Elastic Stack customers. They could also combine it with app monitoring (especially APM modules) and this can be a very focused SaaS service. Whatever is coming down the pipeline from this (integration into Elastic Stack, or continuing as a standalone service like Swiftype - I'm guessing the latter), Elastic is going to be competing in an all new market (software development SaaS tooling). They are already in the enterprise SaaS tooling market now, and against some big names -- Swiftype directly competes with Google! With these new SaaS directions, each opens up all new markets!
It all combines into some fascinating moves by Elastic. I walked into this research project thinking they were a MDB clone, but I now feel Elastic has a much richer story than MDB to me. This SaaS tooling direction took me completely by surprise. [I had here-to-fore under-estimated the Swiftype acquisition more than I should have. And I had never even heard of Insight.io before this research.] I was mightily impressed by Okta after my tech review of them; I am moreso of Elastic. Perhaps due to my closeness to their product, I corralled my thoughts around this company incorrectly. Today, I cannot deny I have a new excitement around the potential here. And, if my financial bet is correct, it's just the beginning of their SaaS moves. TAM potential is completely unknown. Tomorrow they could start or acquire a New Relic or PagerDuty or Everbridge clone. Any SaaS monitoring service is competition to their do-it-yourself on Elastic Stack, but they clearly have their sights on SaaS services built on that for companies that just don't want the additional infrastructure/management of an Elastic Stack cluster.
I think MDB and Elastic are such similar business models, that I'd really like to see a numbers to numbers comparison of MDB and Elastic. Nearly same revenue, nearly same growth [well, maybe... MDB just accelerated this recent Q!], nearly same market cap [then again, MDB just jumped 25%...]. I may move to have nearly equal allocations in them, and start exiting one when it starts faltering in head-to-head comparison of their stats - find the one executing better after a few Qs then move all in to that one. Anyone in the collective want to start tracking and posting a comparison? (Please! I'm too busy pontificating here!)
In closing, I hope I convinced you to revisit any concerns about their open-source strategy. It's two sides of the same battle of keeping their cloud hosting services differentiated against the cloud-vendors' offerings. MongoDB can't be hosted past v3.6, so all new features are proprietary from here. ELK is entirely open and free, but it's the integrated modules that require licensing, and all new features are proprietary from here. Letting companies utilize and embed Elasticsearch into their own products, via that permissive Apache 2.0 license, is what fueled this next phase of Elastic's revenue growth in having these "side-car" SaaS services they've acquired.
Needless to say, I have increased my allocation prior to the publication of this massive missive. I hope you learned something. I sure did, which is why I love this kind of homework.